A guided tour to the Gompertz law of mortality
Human mortality data and the centuries-old quest for a formula to describe it
Here is a question to you: What are the chances of you dying this year?
This is not an easy question, and you may want to avoid it altogether. But regardless of how we might feel about it, our death is (at the minimum) consequential to us and our loved ones. So let’s be fearless and explore the subject with a cool head. Fortunately, a sensible answer (mathematically speaking) to our chances of dying this year isn’t that hard to guess.
Choose a sample of people born in the same year, and — as time goes by — take note of their age at death. Some people will unfortunately die during infancy. Most will face death much older. There is a mathematical expression, known as the Gompertz law of mortality, that give us a reasonable estimate of how many persons of the sample will die each year. The law is usually stated as
where is, loosely speaking, the chance of death at age , and , are constants obtained from experience.
But how did Benjamin Gompertz discover this expression in the first place?
Our tour goes back in history, examines the original papers and data, steps into the shoes of Gompertz and his fellow pioneers, and rederives things from scratch.
We divided this essay into a few foldable sections. Click anywhere on their titles (see below) to expand or hide each their contents. To get your current chances of death, check out the Fast forward to the 2010s section and get your estimates from the interactive charts available there.
The first life tables (1600s)
Mortality has bewildered humankind for aeons, but it was only in the second half of the 17th century that mortality data by age was tabulated for the first time.
The very first life table containing age-at-death statistics of human populations is attributed to John Graunt. His book Natural and Political Observations Made Upon the Bills of Mortality features only crude estimates though. That’s because the primary data he had access to, London’s Bills of mortality (sidenote: Bills of mortality were the weekly mortality statistics in London.) , did not contain any age-at-death information.
A few years later, Edmond Halley — of comet’s fame — gathered rough mortality data in Wrocław, Poland from Caspar Neumann, and published a mortality table in a 1693 paper, An Estimate of the Degrees of the Mortality of Mankind (a).
At the time, the so-called tables of mortality were built to calculate the price of life annuities (sidenote: For those of you (like me) that didn’t know what a life annuity is: it is an insurance product in which the purchaser pays in advance for a sequence of future payments while he or she is still alive.) .
When an insurance company sells a life annuity to someone, it must come up with an expectation for how long the purchaser will live. Insurers are then very interested in predicting their customers’ lifespans as accurately as possible. That’s why they were the ones most that spurred the creation of mortality tables.
Early mortality tables consisted in births and deaths grouped by age for some European localities during the 1600, 1700, and 1800s. Here is a famous one, published by Joshua Milne in 1815:
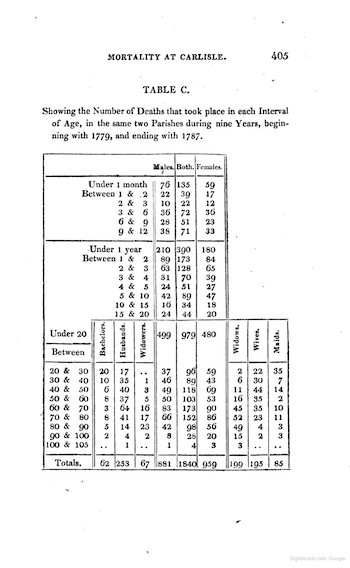
Data from several early tables has been nicely summarized by David Forfar. I plot four of them in the chart below.
For each following data points , the -value represents the number of survivors aged in years. This kind of series is also known as survival curve. To make ours easier to compare, each dataset was rescaled (i.e., divided by the number of people in it) such that for all of them:
A quick look at the chart reveals a few common features among these series:
- A sharp decline between ages and
- A roughly linear decay from to y.o.
- A somewhat accelerated decay afterwards
But beware of jumping into conclusions too fast!
These series were among the first ever tabulated, but they had serious issues. Their sample size, especially for older ages, was quite small. There were methodological and data collection issues as well (sidenote: Read about the issues with data in Makeham 1860, Makeham 1866, Makeham 1867, and Sutton 1884.) .
So early mortality data was rough. But, as soon as the first mortality tables were published, work related to them blossomed among European mathematicians. Besides its practical use in life annuities, the prospects of finding mathematical regularities on human mortality was — and still is — too alluring!
de Moivre’s basic mortality models (1725)
In 1725, the mathematician Abraham de Moivre published the book Annuities Upon Lives with his incursions into the problem of estimating human lifespans (sidenote: de Moivre based his calculations on the table assembled by Edmond Halley in 1693 with data from Wrocław.) .
In his book, de Moivre deliberately converted real lives into fictitious ones. By abstracting from real-world data, he was free to investigate models that were within reach of the Mathematics of his day.
He applied two mortality models to his fictitious populations. They had either:
- An equal number of deaths per year, or
- Equal probabilities of death at each year of age
The first hypothesis is known as de Moivre’s law. An equal number of deaths per year results in an arithmetic progression, and in survival curves following a line. From the previous chart, this simple model seems to fit parts of the early data. In fact, we have just observed a roughly linear decay (from to y.o.).
But you should also recall that the early data was inaccurate. So at the end of the day, his first model has not been very fruitful.
de Moivre’s second model did not reflect real human mortality either. We have later discovered though that it does describe several other phenomena found in nature. So it is useful to explore it further and sharpen our intuition about models.
What does “equal probabilities of death at each year of age” actually mean?
Here’s a way of thinking about it. This hypothesis is as if death were completely independent of age. In such a model, both the young and old die at the same rate. It does not matter if you are young (and usually healthy) or old (and typically frailty), your chances of dying in any giving day are always the same.
In such a hypothetical world, how does the human survival data look like?
They decrease at a constant and age-independent rate. Something very similar to the geometrical progression that happens to atoms in radioactive decay.
To make it visual, let’s consider two example curves of geometrical progressions, with constant rates and , and plot them over the data points that we have seen before:
What to make out of this?
Well, de Moivre did invite Math to the party. But it took a century before the data had become a bit more acceptable to be used as a basis for better models.
Gompertz’s foundational ideas (1820s)
One hundred years after de Moivre’s work, Benjamin Gompertz made a few founding contributions to the quest of a formula for mortality.
Gompertz was a British self-educated mathematician that worked for an insurance company. He published seminal work about a “law of human mortality”. His most famous paper — On the nature of the function expressive of the law of human mortality, and on a new mode of determining the value of life contingencies — came out in 1825. Because of his contributions, he is generally recognized as one of the pioneers of Actuarial science (sidenote: A good, short, readable paper with the historical context of early Actuarial science is Ogborn 1953.) .
His papers touch on different aspects of pricing life annuities. In a way, Gompertz intended to make his job easier. Because calculations were labor intensive at the time, he tried to find general formulae that could fit the data from early mortality tables well enough.
Did he succeed? Not really. Most likely because the data at hand was still of low quality in the 1820s.
Nonetheless, Gompertz ended up making two foundational contributions to the quest of modeling mortality mathematically:
- a conceptual framework for the causes of death, and
- a conjecture for how to account for the effects of aging in mortality
For the framework, here’s what he wrote in 1825:
It is possible that death may be the consequence of two generally co-existing causes; the one, chance, without previous disposition to death or deterioration; the other, a deterioration, or an increased inability to withstand destruction.
He didn’t elucidate what exactly he meant by “chance”, but he did put forward a working model for “deterioration”:
If mankind be continually gaining seeds of indisposition, or in other words, an increased liability to death […] it would follow that the number of living out of a given number of persons at a given age, at equal successive increments of age, would decrease in a greater ratio than the geometrical progression.
In other words, deterioration would increase the susceptibility of humans to death and because of that, survival curves should decline faster at older ages. That rings as true to our present-day intuition. The old does seem to die more frequently than the young.
Gompertz went further and proposed a quantitative model to account for his conjecture of an increasing rate of death as we grow older:
If the average exhaustions of a man’s power to avoid death were such that at the end of equal infinitely small intervals of time, he lost equal portions of his remaining power to oppose destruction which he had at the commencement of those intervals, then at the age his power to avoid death, or the intensity of his mortality might be denoted by , being a constant.
It is fair to say that this is the paragraph that have made Gompertz famous. So it surely bears closer examination!
In Gompertz’s model of deterioration, humans hold two opposing characteristics: the “power to avoid death” and its inverse, the “intensity of mortality.” That is, as one decreases, the other increases. Moreover, the “power to avoid death” would decay in “average exhaustions” over “infinitely small intervals of time.”
The way he mentions these terms suggests that Gompertz intended to cast his concept of “power to avoid death” into what is now known as a continuous random variable. Probability theory was yet to be fully formalized at his time, but he surely was familiar with Calculus and its uses to study rates of change. In fact, in his 1825 paper, Gompertz made extensive use of fluxions — Isaac Newton’s notation for the time derivative — to analyze the rate of change of survival curves.
Gompertz’s paragraph ends with a punchline. He (seemingly out of nothing!) conjectured that intensity of mortality could be expressed by . We shall see (later in our essay) that further progress demonstrated that taking for the intensity of mortality works remarkably well. But where did Gompertz got this nice and tidy closed-form expression from?
Because he offered no explanation in any of his papers, it’s up to us to step into his shoes (and mind), fill in the gaps and rederive it from scratch.
Recreating the steps that Gompertz might have followed
Gompertz was into something with his analyses of rates of change of survival curves. But, as I have just remarked, there were gaps in his exposition. He did not provide a clear definition for what he meant by “intensity of mortality.” Neither did he justified why he picked for its analytic form.
If I were to fill the gaps and recreate step by step what Gompertz might have thought, I would reason as follows (sidenote: I will be “thinking out aloud” step by step. Anyone with familiarity of basic Calculus should follow easily, but bear in mind that I am no mathematician so I won’t be 100% precise.)
First, let be the number of persons alive at age (similarly to the plots of survival curves that we have visited before).
To investigate the rate of change of , we start by computing the absolute decrease of over the -year intervals:
To get a sense of the rate of change on a “more natural” timescale than -year intervals, we then compute the average annual decrease over -year intervals:
Having realized that years is too coarse of an interval (there certainly are tons of “action” happening in-between), we decide to compute the average over intervals of, say, year:
We then recognize that it would be even better if we could generalize this average ratio from year to any . So we write:
To make it easier to refer to it later on, let’s call this expression rate of mortality.
We now pause and think for a few moments about what rate of mortality actually means in plain English. (Do pause and think about it!)
We arrive at the following: it represents “the amount of deaths per unit of time.”
But does it sound like something useful?
It surely does. But then we realize that this metric is not that great for studying human mortality over time. It still has a serious flaw.
As less and less people survive to older ages, we might be misled to think that there are less deaths per unit of time and therefore things are “improving” — while in fact the opposite is true. If there are only persons alive and all die in a year, things seem relatively much worse than if out of persons alive die in a year.
So we reflect a bit more and realize that “the amount of deaths per unit of time considering only the ones that are still alive at the beginning of each unit of time” seems like a good fix for the flaw that we have just identified.
We now wonder about how to translate this new quantity from English words to Mathematics. We then quickly realize that a division by expresses well the conditioning of “considering only the ones that are still alive at the beginning of each unit of time.”
We then try to stress test this new expression. Could this line of reasoning have gone through Gompertz’s mind back in the day?
If we were Gompertz, working at an insurance company, we would be concerned about life annuities. And, for the point of view of an insurer, what are annuities if not: “Given the information that I have about a purchaser (like their age), how much longer should I expect them to be alive and receive their annuities?”
Looking at mortality through this lens seems promising indeed. Not only it would fix the flaw we have identified, but it would also serve well anyone trying to price life annuities. The division of rate of mortality by bakes in, mathematically speaking, the information of how long the purchaser have already lived.
So having found credence to our new expression, we move forward with the math.
First, recall we have just defined rate of mortality in as:
So, while there are persons alive (that is, ) we divide the rate of mortality by :
Now, because by definition for any survival curve, we become aware of the fact that (for any and ) the above expression assumes only non-positive values.
We decide (simply for convenience) to multiply it by so that it only assumes either positive values or zero:
Having reached a seemingly useful expression, we medidate on a name for it, and settle on discrete hazard rate :
Delighted by this result we decide to go even further.
We have already noted that Gompertz mentioned “infinitely small intervals of time” in his conjecture. So we leave the discrete and jump into the realm of limits and differentials. Let’s summon the power of Calculus.
Thus, in present-day notation:
Or, to put it more succinctly,
And call it continuous hazard rate .
A second look at Gompertz’s “intensity of mortality”
I don’t know about you, but I am quite happy with the tidy results that we have just achieved (in the previous section, above).
There is still a remaining question, though. How can we tell if the hazard rate that we have found is the same concept as Gompertz’s “intensity of mortality?”
A way to investigate this is to calculate the hazard rate from the data points available to Gompertz, and then plot them to check if they resemble the exponential of his 1825 paper. If they do, that would be good evidence that he did have our hazard rate in mind and not something else.
So, from above, the discrete hazard rate is
Using it to calculate and plot the hazard rate found in early mortality tables:
So? What do you think? Does it resemble an exponential?
Personally, I cannot fit an exponential with my eyes only.
To make our eyeballing a bit easier, let’s take advantage of the fact that any exponential curve becomes a straight line on logarithmic scale. Let’s switch the -axis to scale. And now that we there, let’s also overlay an exponential function on top of the data points:
Now we can see it! From to years it is visually plausible that the hazard rate from early mortality tables resembles an exponential, .
Of course this is still eyeballing (feel free to download the data and work out the details yourself), but it is now more believable that we have indeed recreated what Gompertz had in mind with his intensity of mortality.
Incidentally, the name intensity of mortality did not stick. In fact, today that same function has multiple names depending on the field you come from. In Statistics, it is hazard rate (or hazard function). In Actuarial science, it is known as force of mortality. In Engineering, it is called failure rate. If anything, so many synonyms attest to the wide applicability of the concept.
Data issues continued to limit progress into the 1900s
The pioneers continued to wrestle with mortality data for several decades over the 19th century. The inadequacy and poor quality of datasets led not only to dead ends in analyses — as the saying goes, garbage in, garbage out — but also to significant financial losses to insurers.
Case in point, the losses the British government took after the Life Annuity Act of 1808. The act, passed in the midst of the Napoleonic Wars, intended to help funding Britain’s war efforts, but it ended up becoming a costly mistake.
Casey Rothschild describes the colorful sequence of events in a 2009 paper, Adverse selection in annuity markets: Evidence from the British Life Annuity Act of 1808:
Prior to the Life Annuity Act of 1808, British government debt consisted almost exclusively of Consols — coupon bonds with infinite maturity. The explicit goal of the act was to replace them with finite-lived debt by allowing individuals to exchange Consols for life annuities. Since Consols were tradable assets, the act effectively opened a life-annuity market.
Annuities sold under this act made twice-yearly tax-exempt payments. The size of these payments depended on the interest rate (the market Consol price) and the age of the annuitant (the nominees). Prices were designed to be actuarially fair; to that end, they were priced to be 2% more expensive than the actuarially fair price implied by the Northampton life table.
Shortly after passage of the Act, there appears to have been a recognition that the use of the Northampton tables was leading to large government losses. Ray D. Murphy writes [in Sale of Annuities by Governments] that it “was wholly unsuitable as a measure of the lower rates of mortality experienced by a self-selected group of annuitants. It was not long before this shortcoming was brought to the attention of the Exchequer.”
You, astute reader, will remember that we have already seen data from the Northampton life table before. (In any case, we will revisit it in a moment.)
Back to Rothschild to learn how the story ended:
In 1823, Parliament finally took active steps to address this perceived mispricing by commissioning John Finlaison to study the mortality experience of the early annuitants. His 1829 report developed a new set of gender-specific life tables based on the observed mortality experience of these nominees. After some debate and a brief suspension of the life annuity program, Parliament determined to resume it with gender-specific pricing based on these new tables (sidenote: I couldn’t get my hands at the full Finlaison tables. They were originally published in 1829 as a Report of John Finlaison, Actuary of the National Debt, on the Evidence and Elementary Facts on which the Tables of Life Annuities are Founded: Ordered by the House of Commons, to be Printed 31 March 1829. The 10-volume set History of Actuarial Science, edited by Steven Haberman and Trevor A. Sibbett and published in 1995, also has the tables (and much more). The set is the historical vade mecum of the field, your local library might have it. Look for the 1829 Finlaison tables in Volume 2.) .
In effect, here is a visual comparison between Finlaison’s data on Government Annuitants and Richard Price’s Northampton table:
As Craig Turnbull writes in his A History of British Actuarial Thought:
Finlaison’s analysis evidenced the profound differences between the government annuity mortality experience and the mortality rates assumed in the Northampton table: for example, at age 60, the Northampton table was implying a mortality rate that was fully double that experienced by the government annuitants!
Beyond the impact it had on government policy, Finlaison’s report was an important milestone for actuarial thought. [… T]he scale and rigour of Finlaison’s analysis was of a different order to these earlier works.
Fast forward to the 2010s
Let’s fast forward to recent times. Although there are still limitations in mortality data for ages above , we have access to data of much higher quality than the pioneers ever had.
The Gompertzian exponential does fit present-day mortality remarkably well. The fit encompasses an age period of approximately years and a range of hazard rates covering four orders of magnitude — from to .
Recall that the Gompertz hazard function is
Here are the constants and , and age periods ( values) that fit the Gompertzian function to recent mortality data from the U.S., England & Wales, and Brazil:
Country | Sex | Age period | ||
---|---|---|---|---|
USA | M | - y.o. | ||
USA | F | - y.o. | ||
E&W | M | - y.o. | ||
E&W | F | - y.o. | ||
Brazil | M | - y.o. | ||
Brazil | F | - y.o. |
You may ask: Where did this table come from? Or, more specifically, how did I find the exponentials that fit the data?
I ran a simple linear regression over recent data provided by the national bureaus of statistics (or equivalent) of these three countries (sidenote: Our data sources are:
- United States Life Tables, 2017 (PDF) by Elizabeth Arias and Jiaquan Xu (CDC/NCHS Life Tables), published in 2019
- English Life Tables No. 17: 2010 to 2012 (PDF) by Jakub Bijak, Erengul Dodd, Jonathan J. Forster, and Peter W. F. Smith, published in 2015
- Tábua completa de mortalidade para o Brasil – 2018 (PDF) by Instituto Brasileiro de Geografia e Estatística (IBGE), published in 2019) .
To convince you (or at least your eyes) that these Gompertzian curves do fit quite well the best public data currently available, let’s plot the official data points and overlay the fitted lines. For males we have:
So, suppose you are a -year old English man.
The “official” data indicates that your chances of dying at this age are . That is, according to death statistics in 2010-2012 in England and Wales, roughly in every English and Welsh men aged died at that age.
The Gompertz law that we fitted to the data predicts that
which is off to the “actual” number by
I don’t know about you, but getting a error from a quick and simple 2-parameter model seems great to me!
Here is the equivalent chart for females:
If you are a -year old American woman, your probability of dying before your th birthday is . In other words, according to U.S. death statistics in 2017, approximately in every American women aged died.
The prediction of our Gompertzian curve is
And the error is
Why is the error much higher for this second example?
It is easy to check visually that the fit is much worse near the start and the end of the age periods — around - y.o. and above the age of .
I plan explore these edges cases in future essays. There are open questions and thought-provoking hypotheses about both ends of the curve.
For instance, youngsters are particularly affected by what is known as “extrinsic” causes of mortality. These are infections, accidents and crime — things that come from “outside.” In fact, if we remove the deaths from extrinsic causes and leave just the ones due to “intrinsic” issues, it seems that the fit to the Gompertz law expands to younger ages very nicely!
As for the mortality of the eldest, due to sparse data (sidenote: For instance, in the paper about ELT 17 Methodology (PDF) the authors write: “Graduation (smoothing) is particularly important at the oldest ages, where exposure numbers are small and data are sparse.”) , it is still not entirely clear to this day if the hazard rate flattens out — or perhaps it even decreases. (I don’t have a strong opinion on the matter yet. I still have several papers on the topic to read and think about.)
I hope you will join me in future essays on these and related topics.
Finally, if you haven’t yet, feel free to scroll up, find you age and eyeball your chances of dying this year. You may not be from any of these three countries. In this case, of course the estimate will be rougher but probably still in the correct order of magnitude.
Appendices
The famous modification proposed by Makeham (1860s)
In the decades following Gompertz’s original papers, it was clear for everyone involved in Actuarial science that the Gompertzian exponential did not fit the data over the entire human lifespan. Despite that, the lure of finding the actual law of mortality continued to motivate the proposal of several other expressions.
In a 1953 paper, On the Nature of the Function Expressive of the Law of Human Mortality, M. E. Ogborn listed some of the attempts that were published in the late 1800s:
Gompertz, himself, suggested in 1860 a formula based on an amalgamation of several of his curves with different constants, and various combinations have been suggested from time to time, together with others of a different type (sidenote: Another example is a formula in Thomas Rowe Edmonds's 1832 book Life Tables, Founded Upon the Discovery of a Numerical Law Regulating the Existence of Every Human Being, Illustrated by a New Theory of the Causes Producing Health and Longevity. Edmonds proposed the use of three exponentials to account for the hazard rate throughout all ages. Each exponential corresponded to one of the “grand divisions of life”: Infancy (birth to 8 years), Manhood (12-55 years), and Old Age (from 55 to end of life). Incidentally, it was Edmonds who coined the term “force of mortality”, used by actuaries to this day.) .
Perhaps the best illustration of the point of view is given by the mathematical formula proposed in 1871 by the Danish mathematician, Thorvald Thiele, to express the rate of mortality throughout the whole of life.
In Thiele’s [7-parameter] formula,
the last term, , is a Gompertz curve to represent old-age mortality and the first, , a decreasing Gompertz curve to represent the mortality of infancy. The middle term, , is a form of the normal curve of error (sidenote: This middle term is likely to account for the hump in mortality experienced by young adults.) .
The contribution to the Gompertz law that ended up being the most famous was proposed by William Makeham in the 1860s. He wrote in a 1866 paper, On the Principles to be observed in the Construction of Mortality Tables:
Mr. Gompertz’s theory of the law of mortality is, that the vital power, or the “power to oppose destruction,” loses equal proportions in equal times; and consequently that the intensity of mortality, which is inversely proportional to this power, is represented by a series in geometrical progression.
It has, however, invariably been found that the ratio of progression, instead of remaining constant throughout the whole period of life, as the theory supposes, is, on the contrary, subject to a slow but continued increase with age; in consequence of which it has been found necessary to change the constants at least once, but generally twice, in the construction of a complete table of mortality.
I venture to think, in a more scientific manner, by supposing the intensity of mortality to be represented by a series not purely geometrical, but consisting of the sum of two terms, the one a constant quantity and the other geometrical. That is, instead of representing the intensity of mortality by an expression of the form we represent it by one of the form .
Makeham then offered the following rationale for his proposal:
From the age of 15, or thereabouts, the normal law of mortality, of which we are in search, is characterized by an increasing progression throughout; the rate of increase, however, being at first very slow, and gradually gaining in rapidity with increased age.
It is this characteristic which renders the formula before described (consisting of a constant combined with an increasing geometrical series) singularly well adapted to represent the law in question from adolescence to extreme old age — a satisfactory proof of which assertion I hope to give on a future occasion, when I propose also to examine the results of an extension of the formula to all periods of life.
Makeham’s “satisfactory proof” on “a future occasion” came out the next year. In 1867 he published another paper — On the Law of Mortality — further expanding on his hypothesis.
He used data from five U.K. mortality series from the 1800s to support his argument. To check it out visually, let’s plot the data points from all five data series as presented by him:
Now, let’s overlay two curves on top of his data points: a Gompertz’s exponential (in light gray) and the Makeham’s proposed modification (dark gray).
From the chart below, it is pretty clear that Makeham’s modification did improve the fit to the data available at his time:
Part of the appeal of Makeham’s modification is that it adds just one additional parameter to the expression (for a total of three). From a modeler standpoint, too many parameters isn’t good. After all, given enough parameters, any dataset can be fitted (sidenote: John von Neumann is often quoted having said that: “With four parameters I can fit an elephant, and with five I can make him wiggle his trunk.” For an actual elephant-fitting function, check out this cool 2010 paper, Drawing an elephant with four complex parameters.) .
As much as it was an improvement, the Gompertz-Makeham law is not the ultimate law of mortality. Makeham avoided infancy and childhood altogether in his 1867 paper. In fact, he wrote at the time:
I must postpone to a future opportunity the examination of so interesting and important a subject as the mortality of infancy and childhood.
(No harsh judgement. We also postponed the examination of infancy to a future essay.)
Survival function in terms of hazard rate
We have already have in terms of from (see below). Let’s work out a few more steps to express in terms of , and come full circle.
Recall that
By the Fundamental theorem of Calculus, we can write
When thinking about how to solve the integral at right-hand side, we recollect that the natural logarithm somehow resembles it.
Moreover, because we defined , we can summon the Chain rule as:
And insert this result into the previous expression
By the Fundamental theorem of Calculus again, we have
Thus,
Finally, by the definition of logarithms
And there you have it, expressed in terms of .
This is especially useful for when the hazard rate is known and we intend to find its corresponding survival curve. That is exactly what we plan to do in a future interactive essay. (Hopefully soon.)